The Evolution of Business through Labeling Tool Machine Learning
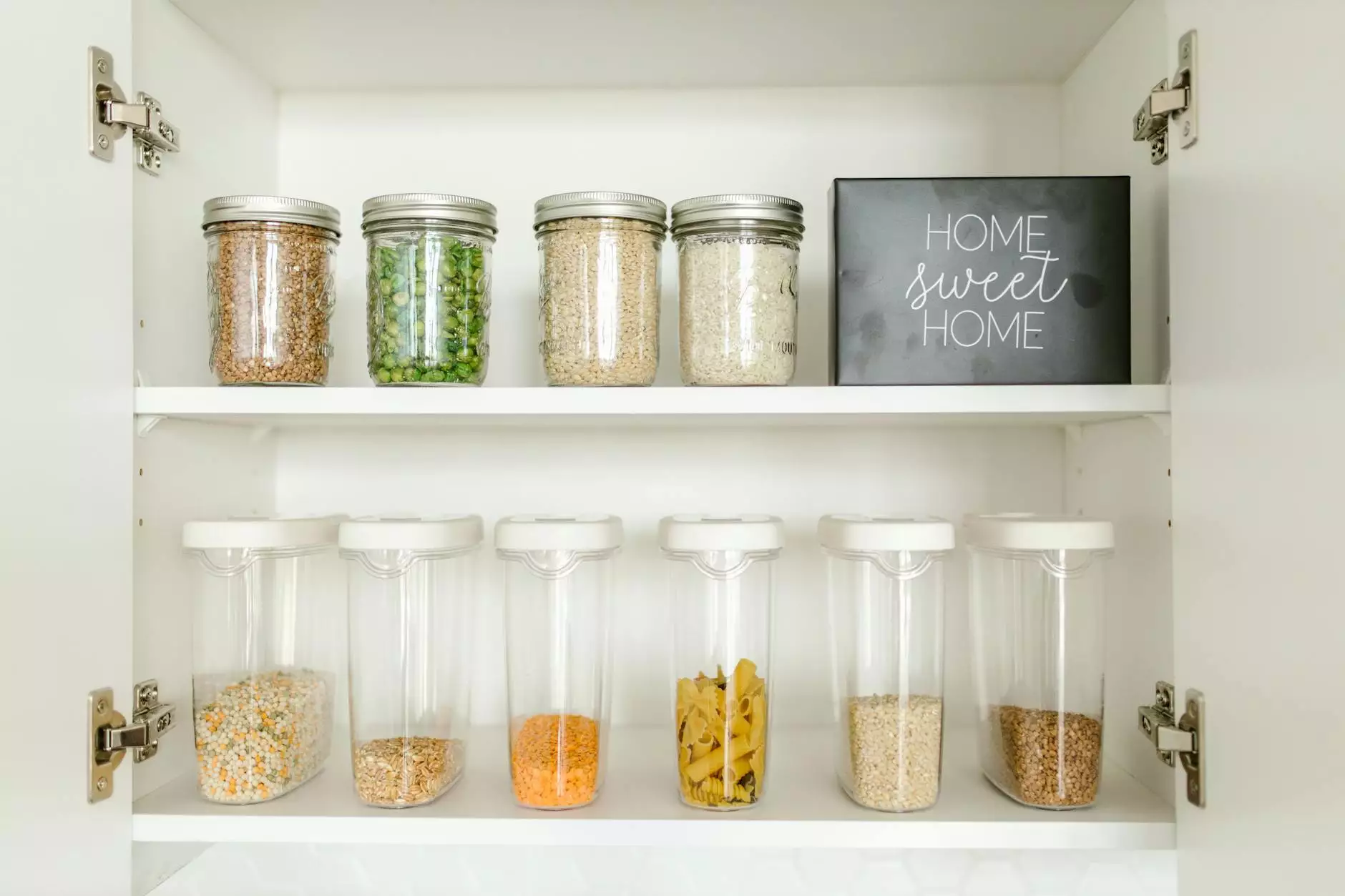
The modern business landscape is ever-changing, with an increasing emphasis on data-driven decision-making. As companies harness the power of data, the labeling tool machine learning emerges as a critical component in ensuring that this data is not only accurate but also actionable. This article delves deep into the significance of labeling tools in machine learning, their applications, and how they can revolutionize various business operations.
Understanding Machine Learning and Data Annotation
Before diving into labeling tools, it's essential to grasp what machine learning is. Machine learning is a subset of artificial intelligence that allows systems to learn from data, improving their accuracy without being explicitly programmed. At the heart of machine learning lies data annotation – the process of labeling data to train algorithms effectively.
Importance of Data Annotation
Data annotation is critical because:
- Enhancement of Algorithm Performance: Annotated data helps algorithms recognize patterns and make predictions more accurately.
- Quality Insights: Businesses glean actionable insights through properly labeled data, driving strategic decisions.
- Time Efficiency: Automation facilitated by machine learning reduces the time spent on manual data processing.
The Role of Labeling Tools in Machine Learning
A labeling tool streamlines the data annotation process, ensuring that large datasets are labeled quickly and accurately. The significance of using robust labeling tools in machine learning cannot be overstated. Here’s why:
1. Scalability
As businesses grow and data requirements escalate, the capability to manage large volumes of data becomes paramount. Labeling tools are designed to handle large datasets efficiently without sacrificing quality.
2. Cost-effectiveness
Employing machine learning with a reliable labeling tool significantly reduces costs related to data processing and annotation. The automation of repetitive tasks means that companies can allocate their resources to more strategic areas.
3. Accuracy and Consistency
Human errors can compromise data quality. Labeling tools help mitigate such risks by providing standardized processes that ensure consistent labeling, ultimately improving the accuracy of the machine learning models.
4. User-friendly Interfaces
Many modern labeling tools come with intuitive interfaces, making them accessible to non-technical users. This democratization of technology means that more employees can contribute to data labeling efforts, fostering a collaborative environment.
Types of Labeling Tools
There are several types of labeling tools, each serving distinct purposes. Here’s a breakdown:
1. Image Annotation Tools
Used primarily in computer vision tasks, these tools help label images with bounding boxes, polygons, and more. This type of labeling tool is essential for training models to recognize objects and patterns in visual data.
2. Text Annotation Tools
These are pivotal for natural language processing (NLP) applications. They facilitate the labeling of text data by identifying entities, emotions, or sentiments, enabling models to understand language nuances.
3. Audio and Video Annotation Tools
In multimedia applications, these labeling tools help annotate audio files or videos, marking specific sounds, dialogues, or actions, which are valuable for training models in speech recognition and video analysis.
Case Studies: Labeling Tools in Action
1. Healthcare Applications
In the healthcare sector, labeling tool machine learning can enhance diagnostic accuracy. For instance, radiology departments use image annotation tools to label medical images, aiding in the identification of tumors. By training machine learning models with accurately labeled data, healthcare providers improve patient outcomes through more precise diagnostics.
2. E-commerce and Retail
In the e-commerce industry, businesses utilize text annotation tools to analyze customer reviews and feedback. By labeling sentiments attached to product reviews, retailers can tailor their offerings and improve user satisfaction, leading to increased sales and customer loyalty.
3. Autonomous Vehicles
Self-driving technology relies heavily on computer vision. Labeling tools provide the necessary framework to annotate images and videos from various sensors onboard, with the goal of recognizing road signs, pedestrians, and other vehicles. This precision in labeling is crucial for the safety and reliability of autonomous systems.
Choosing the Right Labeling Tool for Your Business
Selecting a labeling tool involves considering several factors:
1. Use Case
Your choice should align with your specific use case. Whether you need tools for image, text, or audio annotation, ensure the tool fits your business requirements.
2. Integration
Choose tools that integrate seamlessly with your existing tech stack. Ideally, labeling tools should support APIs and support data import/export functionalities to maintain workflow efficiency.
3. User Support
Opt for tools with strong customer support. Reliable support can be a lifeline when navigating technical challenges or seeking to optimize your labeling processes.
4. Cost
Evaluate the total cost of ownership, including subscription fees, training costs, and any additional expenses that may arise from implementing the tool in your organization.
Future Trends in Labeling Tools and Machine Learning
The future of labeling tool machine learning is bright, characterized by innovative trends that promise to enhance efficiency and accuracy:
1. Automation and AI-enhanced Labeling
As artificial intelligence continues to evolve, we can expect more automated data labeling solutions. Tools that utilize AI to expedite the labeling process while maintaining high-quality standards will become increasingly prevalent.
2. Crowdsourced Labeling
Crowdsourcing offers a solution for businesses facing large-scale annotation challenges. By leveraging a distributed workforce, companies can annotate vast datasets swiftly, although this requires robust quality control measures.
3. Enhanced Collaboration Features
Future tools will likely focus on facilitating better collaboration among data scientists, subject matter experts, and labelers. Features that support real-time feedback and iterative improvements will enhance the overall data labeling process.
Conclusion: Embrace the Change with KeyLabs.ai
The integration of labeling tool machine learning into business operations signals a profound shift towards data-driven insights and automation. KeyLabs.ai stands at the forefront, providing state-of-the-art Data Annotation Tools and Data Annotation Platforms to meet the diverse needs of modern businesses. By adopting robust labeling tools, you not only enhance your data quality but also empower your team to make informed decisions that drive success.
As we move forward, businesses that harness advanced labeling tools and machine learning capabilities will not only adapt but thrive in an increasingly competitive environment. Embrace this technological advancement today and transform your business strategy with KeyLabs.ai.